AI: The New Frontier of Class Struggle
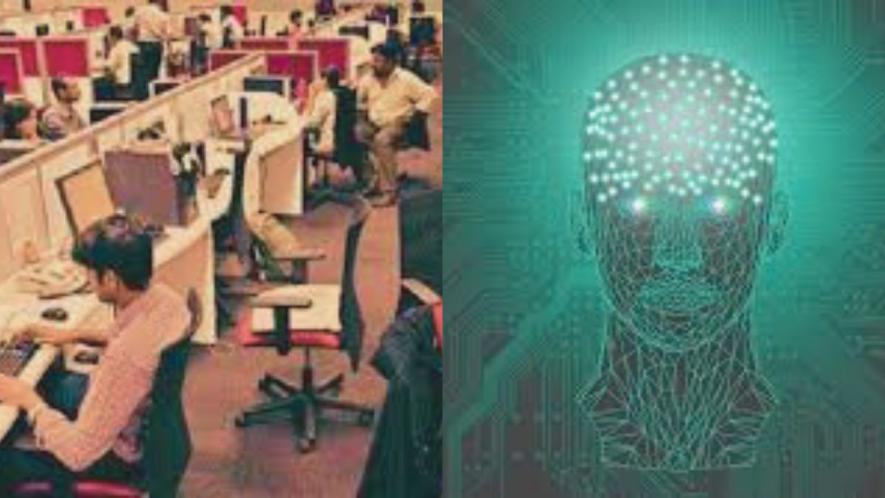
File Image
Writing on the “Future Results of British Rule in India” in 1853, Karl Marx remarked:
“All the English bourgeoisie maybe be forced to do (regarding laying down of railway lines and setting up industries in India) will neither emancipate nor materially mend the social condition of the mass of people, depending not only on the development of the productive powers, but on their appropriation by the people. But what they will not fail to do is lay down the material premises for both.”
In simpler terms, the two factors that he outlines for the masses’ emancipation can be broken down into technology (productive powers) and its ownership by them (appropriation by the people). While this theorisation was for the colonialist stage of capitalism, it offers a potent framework for the new era of artificial intelligence- or AI-powered capitalism and the policy measures that can harness its power for the commons.
The advances in AI, with the LLMs (large language models) that ChatGPT and others have brought up, have resulted in new systems of production that will reduce labour costs by automating a lot of low-level intellectual labour tasks. As such, there is a mad scramble among all corporations for utilising it as effectively as they can. Companies are pushing more and more resources into both researching the feasibility as well adoption of AI into their production chains.
In the mainstream discourse on AI, the relation of it to labour remains largely out of scope or marginalised. It is, however, of paramount importance to understand the transformation that it has undergone.
A key point regarding the mechanisms of AI is to understand that across all major machine learning models, especially LLMs, there is a feedback loop that instructs the model if it is correct or wrong. Here, the basis of this mechanism is that there must be something to feed itself on, to correct itself. That is where human labour comes into the picture.
All these AI models must utilise copious amounts of human-generated data, scraped (a lot of times illegally) from the web. This data ranges from answers given on Stack Overflow, to illustrations posted on DeviantArt, to even photographs taken from scouring Facebook.
An obfuscation occurs when we label these solely as “data”, as the source that creates all this is labour. More specifically, it is the intellectual labour of the masses of people that is being supplied to power AI. At the same time, the ownership of these labour-powered AIs rests in the hands of the mega corps. who also exploit the commons (water and electricity) for training them.
When we proceed from this understanding, it is easy to grasp that labour relations are now being redefined: that machine learning is doing to intellectual labour what machines did to physical labour. Thus, it stands to follow that labour policies must be formulated to safeguard workers’ rights.
There is a peculiarity to this intellectual labour: at the time of original creation, say, when someone answers a query on Reddit regarding the difference between two Dragon Ball Z characters, it is merely communication and partaking in the public square. Yet, when this very conversation is scraped and read into the LLMs, and when this is sold to consumers and corporations, that conversation gets a value assigned to it as it is ultimately aided in value creation. And yet, none of the original creators are ever compensated for it.
This situation is more evident in image generation AIs, which have been noticed to produce images in the styles of certain digital artists and in mimicking them quite well, thereby making it blatant that they were trained in their artwork without consent or compensation.
As such, AI has intruded upon a class of people who were, up until now, largely regarded as safe from mechanisation-related layoffs and job losses. AI corporations have used their knowledge and labour to drive them off the market.
Alongside this group of people, there are those involved directly in producing intellectual labour for AI. The well-known maxim of AI being “Actually Indian” points to the large-scale utilisation of cheap labour from semi-peripheral and peripheral nations for processes of data labelling and model training. In this, the data output by AI is checked by these workers as being correct or wrong, thereby explicitly helping it get better trained.
Taken all together, these two groups of workers constitute the gamut of labour production involved in machine learning. They can be classified as being direct labourers (the latter group, being explicitly involved in the learning process) and indirect labourers (creating knowledge that becomes labour once AI utilises it in the form of training data).
For labour policies to be effective, both sets of workers must be henceforth recognised in law. The question now is: how do we go about doing this? For without a perception shift, it would be immensely difficult to gain recognition in law, especially for indirect labour.
A solution comes from Anupam Guha (Professor, IIT, Bombay), who proposes that AI be treated as a “public good”:
“There’s a need to challenge “free-market” fundamentalism and initiate international cooperation on AI policy, and start large, public funded, and distributed AI research, AI public-works, and AI-centric education,” he says.
Extending this model over data-as-labour, direct and indirect labour, and other anti-monopolist methods, AI policy-making acquires a solid base upon which to formulate its response to the current market trend of “blitzing”, in which regulators and policy-makers play catch-up to corporations’ pace of development that frequently involves shady to downright exploitative practises.
A New Labour Contract
The labour policy must recognise indirect labour that is specifically procured from public sources. This would indicate that datasets so generated cannot be made proprietary at all. To this end, the concept of “public data” must be advanced, in which datasets cannot be profited from if they aren’t made publicly available and open source. Companies failing to do so must have their data banks audited and assessed for violation of intellectual property and labour.
The creation of an intellectual labour bureau under existing national labour organisations is recommended. This bureau can then take actions, such as fines appropriate for violation, force the companies to make the data public, and could outright recommend termination of their AI models. All public data must also be open to being flagged by the public.
Another aspect that this bureau could investigate is assessing the actual work done by direct labourers. In the works of translation, for instance, companies have relegated translators to being merely “spell-checkers” who correct the mistakes made by AI. As such, the remuneration rate has been drastically cut for the worker. However, what happens in a lot of cases is that the translator must spend time completely re-writing sentences that the AI has spit out, causing the work done to be equivalent to the work they would do under the pre-AI contract.
Alongside the work done by “Actually Indian” workers as mentioned above, the bureau could thus recommend a better labour contract after taking all these inputs into consideration.
Empowering the Masses
While the recommendation above contended with a perception shift, there must also be a paradigm shift in the production of AI models. Writing on the method to “democratise AI”, Urvashi Aneja (Founder, Digital Futures Lab) suggests “small AI” against the stale empiricism that AI methodology is racing toward.
She advocates a theory of change, in which causal mechanisms and domain expertise is given importance over hoarding massive amounts of data. In this way, the data required could be drastically cut down, and cross-disciplinary collaboration would take place, making the machine learning process democratic in terms of both peoples employed and experiences noted.
A government initiative championing “small AI” could potentially unleash the power of AI to a wider group of people. Implementing this under government-aided AI research facilities that could also take the form of AI co-operatives or PSUs (public sector undertakings) would ultimately lead to the masses gaining ownership for themselves.
Thus, increasing the ambit of workers for AI would also bring about utilisation of it for use in agriculture, water supplies, urban planning, healthcare, and even in optimising grain supply routes under the public distribution system.
The writer is an IT worker and a freelancer interested in the intersections of tech and society. The views are personal.
Get the latest reports & analysis with people's perspective on Protests, movements & deep analytical videos, discussions of the current affairs in your Telegram app. Subscribe to NewsClick's Telegram channel & get Real-Time updates on stories, as they get published on our website.